Research
Research in the Action Lab is dedicated to the experimental and theoretical study of human motor control and learning. Our experiments collect kinematic and kinetic data, complemented by electromyographic and encephalographic data. Physical models of the task and their mathematical analysis provide understanding task solutions as basis for insight into human control.
Nine major themes of research investigate the acquisition, control, and retention of motor skills.
From Action to Interaction; Complex Object Manipulation: This research examines human interaction with complex objects, in particular complex objects with internal dynamics. Using the exemplary task of carrying a cup of coffee we examine how interaction ensures predictability of the object dynamics.
Assessment of Stability in Complex Human Movements: This research develops mathematical tools to assess dynamic stability in human trajectories that do not map onto known attractors.
Control of a Bullwhip – Human Dexterity As It Is: We have designed a naturalistic task of hitting a target with the whip which requires whole-body coordination and interaction with a (potentially chaotic) complex object. The study investigates how a complex movement is orchestrated in humans and how the physics of the whip contributes to acquisition of this skill.
Autism as an Impairment in Prediction: Complementary to our work on predictability in human object control, this line of research examines whether the motor coordination challenges in individuals with autism are due to difficulties in prediction.
The MAGIC Table: A Motion-Analysis Device for Upper-Limb Research: An extension from the research on complex object control that is conducted in a virtual environment, this line of study developed a real device that mimics the simplified task model. The portable device is also used for the study of recovery in stroke patients.
Influence of Physical and Human Support on Balance under Challenging Conditions: We study how healthy humans maintain balance when standing on a narrow beam supported by canes or other humans.
Dynamic Primitives; A Fundamental Hypothesis on Motor Control: We test the hypothesis that complex human actions are generated by employing dynamic primitives: discrete and rhythmic movements, and impedance. These basic units are combined to control complex interactive behavior.
Variability and Stability in Skill Acquisition: We developed a new approach that quantifies acquisition and performance in motor skills by decomposing performance variability into three components: Tolerance, Noise, Covariation. These concepts serve to design novel interventions to improve and accelerate skill acquisition.
Movement as a Vital Sign in Preterm Infants: The development of movement preterms in preterm infants is hypothesized as an important biomarker, which, similar to heart rate and blood pressure, can reveal functional integrity of the nervous system. We develop novel methods to extract movement bouts for the quantitative assessment of cardiovascular and respiratory activity.
From Action to Interaction: Complex Object Manipulation
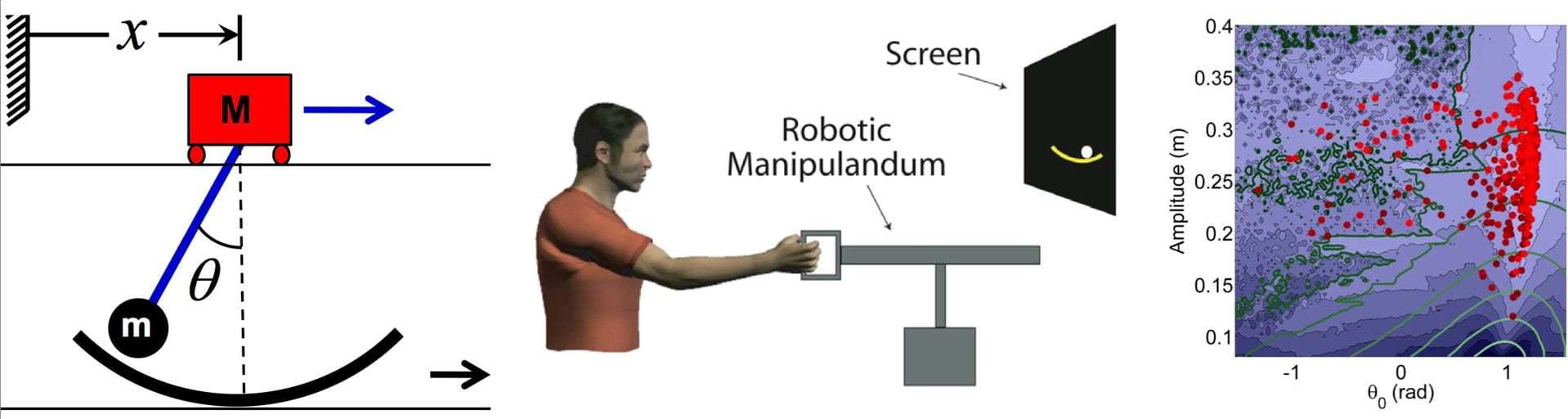
The manipulation of complex objects, or tool use, is ubiquitous in everyday life and has given humans their evolutionary advantage. Such object interactions become particularly intriguing when the objects themselves have internal degrees of freedom and add complex dynamics to the interaction. For example, when bringing a cup of coffee to one’s mouth, the coffee is only indirectly controlled via moving its container. The dynamics of the sloshing coffee creates complex interaction forces that the person has to take into account during control. To gain insight into the control mechanisms underlying this remarkable skill, this research examines the strategies that humans choose when manipulating an object with complex internal dynamics, mimicking a cup of coffee.
Using the cart-and-pendulum model implemented in a virtual environment as a cup containing a rolling ball, our research studies the strategies that people choose when manipulating this object via a robotic manipulandum. A first study examined point-to-point movements, quantifying how the safety margin changes with practice as a function of time constraints [1]. The same task sensitively shows how older people have larger safety margins, i.e., a higher risk of spilling [2]. When subjects move the “cup of coffee” in continuous rhythmic fashion, the complex nonlinear dynamics comes to the fore [3, 4]. Analysis of forces show that subjects seek strategies that make the interaction object more predictable, avoiding chaos. A recent study examined how subjects may prepare, or initialize, the complex object to achieve predictable states faster with shorter transients. Results showed that subjects indeed converged to a small subset of initial conditions that shortened the start-up transients and reached more predictable states [5].
Selected Publications
- Hasson, C.J., Shen, T., & Sternad, D. (2012). Energy margins in dynamic object manipulation. Journal of Neurophysiology, 108, 5, 1349-65.
- Hasson, C.J. & Sternad, D. (2014). Safety margins in older adults increase with improved control of a dynamic object. Frontiers in Aging Neuroscience, 6:158.
- Nasseroleslami, B., Hasson, C.J., & Sternad, D. (2014). Rhythmic manipulation of objects with complex dynamics: Predictability over chaos. PLoS Computational Biology, 10(10), e1003900.
- Maurice, P., Hogan, N., & Sternad, D. (2018). Predictability, effort, and (anti-)resonance in complex object control. Journal of Neurophysiology, 120, 2, 765-780.
- Nayeem, R., Bazzi, S., Hogan, N., & Sternad, D. (2020). Transient behavior and predictability in manipulating complex objects. IEEE International Conference on Robotics and Automation (ICRA 2020), Paris, France, May 31-June 4.
- Nayeem, R., Bazzi, S., Sadeghi, M., Razavian, R. & Sternad, D., 2023, ‘Multi-Modal Interactive Perception in Human Control of Complex Objects’, International Conference on Robotics and Automation (ICRA 2023). DOWNLOAD
Assessment of Dynamic Stability in Complex Human Movements
Selected Publications
- Hasson, C.J., Shen, T., & Sternad, D. (2012). Energy margins in dynamic object manipulation. Journal of Neurophysiology, 108, 5, 1349-65.
- Hasson, C.J. & Sternad, D. (2014). Safety margins in older adults increase with improved control of a dynamic object. Frontiers in Aging Neuroscience, 6:158.
- Nasseroleslami, B., Hasson, C.J., & Sternad, D. (2014). Rhythmic manipulation of objects with complex dynamics: Predictability over chaos. PLoS Computational Biology, 10(10), e1003900.
- Maurice, P., Hogan, N., & Sternad, D. (2018). Predictability, effort, and (anti-)resonance in complex object control. Journal of Neurophysiology, 120, 2, 765-780.
- Nayeem, R., Bazzi, S., Hogan, N., & Sternad, D. (2020). Transient behavior and predictability in manipulating complex objects. IEEE International Conference on Robotics and Automation (ICRA 2020), Paris, France, May 31-June 4.
- Nayeem, R., Bazzi, S., Sadeghi, M., Razavian, R. & Sternad, D., 2023, ‘Multi-Modal Interactive Perception in Human Control of Complex Objects’, International Conference on Robotics and Automation (ICRA 2023). DOWNLOAD

Physical interaction with everyday objects and tools is intriguing when the objects have complex (underactuated, nonlinear, chaotic) dynamics. As described above, when bringing a cup of coffee to one’s mouth, the coffee is only indirectly controlled via moving the cup. The dynamics of the sloshing coffee creates complex interaction forces that the person has to take into account during control.
How do humans manage such complex interactions? Full reliance on a complex internal model of the object is unlikely, and compensation for inadequate prediction and noise via feedback control is problematic due to the relatively long delays in the neuromotor system. We hypothesized that humans seek to stabilize their trajectories, thereby obviating the need for feedback corrections and simplifying the required internal model.
Existing methods for stability assessment in human movements either linearize the system around a fixed point and calculate the Lyapunov exponents (applicable tasks include postural control and stick balancing), or look at steady-state locomotion and calculate the Floquet multipliers. The physical interaction tasks we are interested in are transient in nature, and hence a trajectory-based method of stability assessment is required. We propose that contraction analysis, a differential form of stability analysis developed for nonlinear dynamical systems, is an appropriate tool.
Using the cart-and-pendulum model implemented in a virtual environment as a cup containing a rolling ball, we assessed the stability of the trajectories when manipulating this object in the face of perturbations. Experimental results showed that subjects exploited stability to overcome the perturbations: subjects moved through contraction regions to attenuate perturbations and make the dynamics more predictable. In more recent work, we leveraged the framework of control contraction metrics and showed that subjects actively sought to exponentially stabilize their trajectories directly before and after the perturbations [1, 2, 3, 4].
Selected Publications
- Bazzi, S. & Sternad, D. (2020). Human control of complex objects: towards more dexterous robots. Advanced Robotics, 34, 17, 1137-1155.
- Bazzi, S., Sternad, D. (2020). Robustness in human manipulation of dynamically complex objects through control contraction metrics. IEEE Robotics and Automation Letters, 5, 2, 2578-2585.
- Bazzi, S., Ebert, J., Hogan, N., & Sternad, D. (2018). Stability and predictability in human control of complex objects. Chaos: An Interdisciplinary Journal of Nonlinear Science, 28(10), 103103.
- Bazzi, S., Ebert, J., Hogan, N., Sternad, D. (2018). Stability and Predictability in Dynamically Complex Physical Interactions. IEEE International Conference on Robotics and Automation (ICRA 2018), 5540-5545.
Autism as an Impairment in Prediction
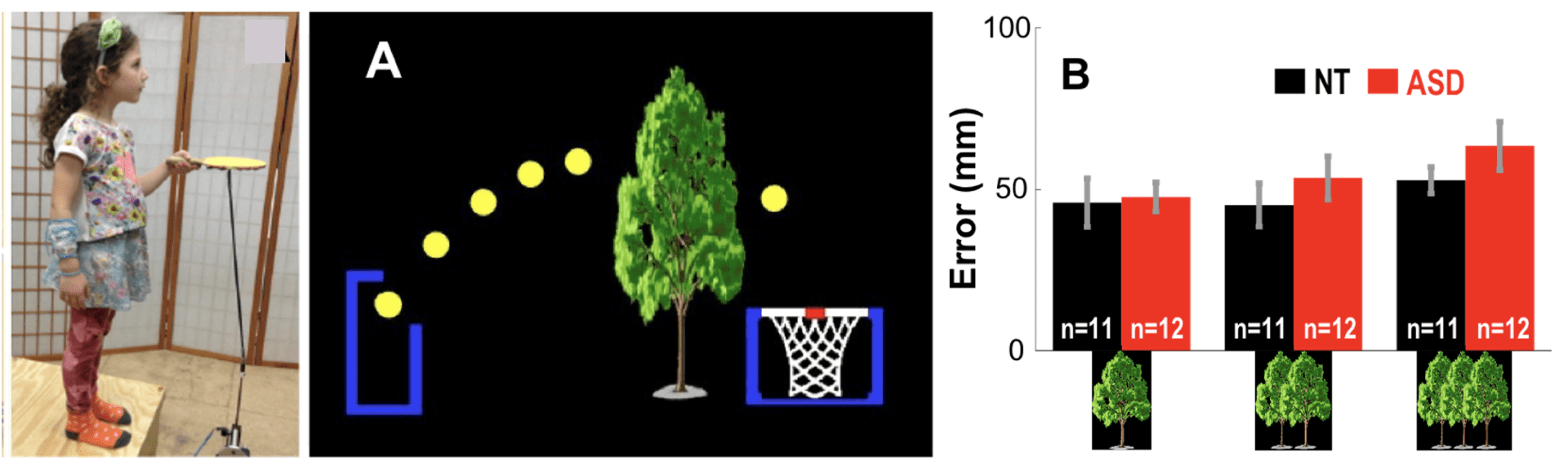
Autism spectrum disorder (ASD) is a complex neurobehavioral disorder, defined by core deficits in social communicative interaction and restricted, repetitive behaviors, frequently co-occurring with impairments in cognition, language and motor function. Individuals with ASD often exhibit difficulties in motor coordination, especially when interacting with dynamic objects, like catching a ball or avoiding approaching cars. Motivated by a theory developed by Sinha et al. (2014), we hypothesize that individuals with ASD have difficulties with dynamic environments due to impaired predictive abilities. Using motor skills that involve catching a ball, a series of experiments selectively quantify impairments of prediction in naturalistic and virtual environments with controlled predictive and coordinative challenges. We compare performance of ASD and neurotypical children when catching a real ball, launched with controlled trajectories. Control tasks evaluate the contribution of potential coordinative challenges, such as postural balance, reaction time, and proprioception [1].
Another series of experiments in a virtual environment compare neurotypical children with ASD in different games with intercepting and bouncing a ball. The virtual set-up affords titrating challenges, such as occlusion and the time available for prediction, while simplifying the coordinative challenges. The behavioral measures are correlated with an extended set of clinical measures of autism severity and used as covariates to interpret experimental performance. Performance of children is compared to that of a cohort of adolescents to examine developmental change. Such understanding is significant for identifying early biomarkers and interventions that address an underlying neurocognitive mechanism, i.e., prediction, and not merely the manifestations of the heterogeneous phenotype [2].
A recent study on a large cohort of ~400 participants in an outreach activity at the Museum of Science in Boston used the same testbeds to test predictive abilities over the lifespan. Results revealed that predictive abilities reach the level of adulty performance at the age of 12 years [3].
Selected Publications
- Bond, S., Park, S-W., Russo, M., Cardinaux, A., Sinha, P., Sternad, D. (2021). Predictive impairments in motor skills in children with autism spectrum disorder. Global Connectome Virtual Conference of the Society for Neuroscience. January, 11-13.
- Park, S-W., Cardinaux, A., Guo, D., Ben-Ami, S., Denna, L., Sinha, P., & Sternad, D. (2019). Predictive motor impairments in children with autism spectrum disorder: Evidence from kinematics and muscle activity. Conference of the International Society for Autism Research (INSAR), Montreal, May 1-4.
- Park, S-W., Cardinaux, A., Guo, D., Sinha, P., & Sternad, D. (2019). Developmental aspects of predictive motor abilities. 49th Annual Conference of the Society for Neuroscience, Chicago, IL, October 19-23.
The MAGIC Table – From the Virtual Cup Task to a Real 3D Testbed
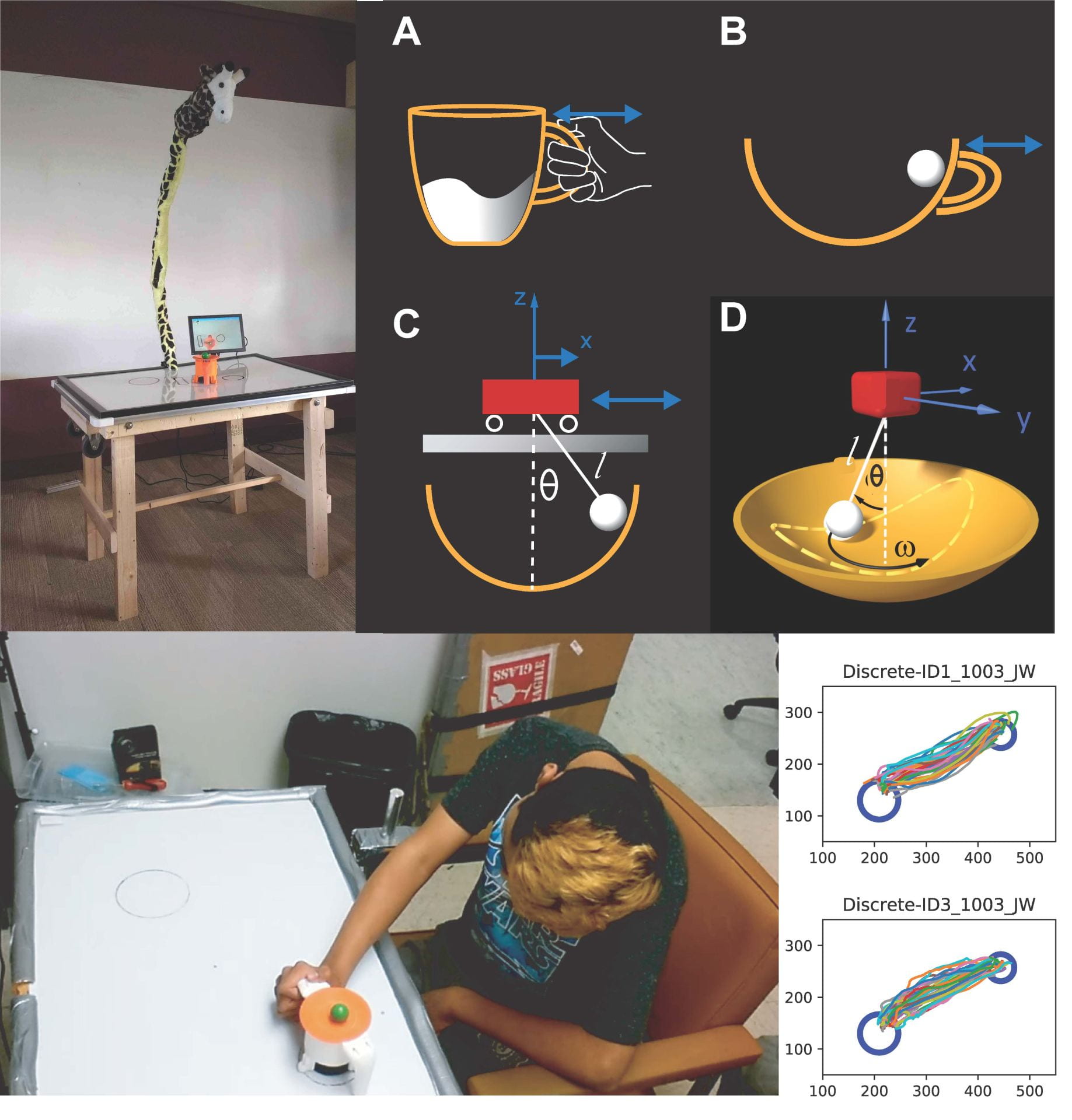
An essential part of clinical care for individuals with motor disorders is to accurately assess motor behavior to inform and evaluate medical or therapeutic treatment. One of the challenges for such assessments is to define sensitive and quantitative metrics that can be readily obtained in clinical practice and that go beyond descriptive outcome or error measures. The current standard tools for motor assessment are the clinical scales which, though useful, rely on subjective assessments and questionnaires that are vulnerable to test/retest reliability, nonlinearity, and ceiling or floor effects. On the other hand, conventional motion capture systems that provide precise measurements are not suited for clinical or home settings, as they are expensive and require attachment of sensors to the body. Further and importantly, assessments need to go beyond pure outcome measures and shed more light on the coordinative issues of the tested individual [1]. Therefore, it is desirable to develop assessments that are better grounded in scientific paradigms.
The MAGIC Table was developed to fulfil these goals. The MAGIC Table affords quantitative assessment of motor coordination in non-laboratory settings, both for individuals with upper-limb movement disorders and for basic research questions. The experimental task is motivated by the everyday activity of moving a “cup of coffee”. Sitting at a table subjects moves a 3D-printed cup with a ball rolling inside to negotiate targets that can be drawn on the table surface. The ball rolling inside the cup enhances the challenge by introducing additional dynamics. An adjustable magnet lightly attracts the cup to the surface to maintain movements in the horizontal plane so that a single video camera above the table can track real-time kinematics. A custom-developed, color-based computer-vision algorithm can record both the cup and the rolling ball. Based on the real-time 2D cup coordinates, audio-visual feedback about performance can be delivered for motivation. The feasibility of using this device in clinical diagnostics has been demonstrated on two neurotypical children and also three children with upper-extremity impairments in the hospital, where conventional motion-analysis systems are difficult to use [2].
This experimental set-up extends from our research on the control of dynamically complex objects, the cup-and-ball task [3]. Simplified to the 2D virtual interface, this basic research has examined how subjects deal with interaction forces that are practically unpredictable. Several studies have demonstrated that subjects make these interactions more predictable [4]. The MAGIC Table represents the first step to the 3D rendering, here in a real environment.
Selected Publications
- Sternad, D. (2015). From theoretical analysis to clinical assessment and intervention: Three interactive motor skills in a virtual environment. IEEE Virtual Rehabilitation Proceedings (ICVR), International Conference on, Valencia, Spain, June 9-12, pp. 265-272.
- Sohn, W. J., Sipahi, R., Sanger, T. D., & Sternad, D. (2019). Portable Motion-Analysis Device for Upper-Limb Research, Assessment and Rehabilitation in Non-Laboratory Settings. IEEE Journal of Translational Engineering in Health and Medicine, 7, 1-14.
- Hasson, C.J., Shen, T., & Sternad, D. (2012). Energy margins in dynamic object manipulation. Journal of Neurophysiology, 108, 5, 1349-65.
- Nasseroleslami, B., Hasson, C.J., & Sternad, D. (2014). Rhythmic manipulation of objects with complex dynamics: Predictability over chaos. PLoS Computational Biology, 10(10), e1003900.
Control of Balance under Challenging Conditions
In our daily life it is critical to maintain balance, not only while standing, but also when walking. To reduce the risk of falling, frail individuals often use a cane or a walker, or hold onto hand rails whenever possible. In contrast, trained humans, like ballet dancers or gymnasts, can achieve astonishing balancing performance. For example, during a pas de deux a ballerina can hold a pose on the tip of one foot by only lightly touching the hand of her partner, or even all by herself. What are the mechanisms by which the CNS can control our balance in such unstable conditions? What is the role of the hands and the arms in challenging balancing tasks?
To better understand neuromotor control of human balance during walking, we studied unconstrained walking on a narrow beam. Using dimensionality analysis of the high-dimensional patterns found that more regularities were found when angular momentum of body segments was examined compared to joint angle kinematics [1]. We also examined how unimpaired individuals maintain balance with the help of rigid footwear. Subjects again walked on a narrow beam either barefoot or wearing rigid flat soles that eliminated movement of the joints in the foot. Variability of the center of mass clearly showed that rigid footwear increased the contact surface that allowed the center of pressure more room to stabilize upright position. However, this effect proved to be entirely mechanical as it was not transferred when walking on barefoot again [2].
In an ongoing series of studies, we assess postural sway of subjects when they supported themselves by one or two canes. To challenge balance, healthy human subjects stood on a narrow beam and were instructed to exert force onto their canes. Our results show that only touching the ground provides maximum assistance, more than higher forces, probably due to the instability of the cane, i.e., an inverted pendulum [3]. Another series of simulation study examined stability of an inverted double-pendulum, comparing simple controllers [4, 5]. Analysis of magnitude and direction of ground reaction forces at different frequencies teased apart relative contributions of biomechanics and control [6].
A current study compares passive cane support with active support from another human. Participants held a robotic arm that provided passive support with different degrees of mechanical stability. In the active support-condition a second individual provided support by holding the same robotic arm. Participants included professional ballet dancers who are trained to maintain or give balance by light touch.
News: see here!
Selected Publications
- Chiovetto, E., Huber, M.E., Sternad, D., & Giese, M.A. (2018). Angular momentum organization for dynamic balance control during walking on a narrow beam. Scientific Reports, 8, 1, 6362. Doi: 10.1038/s41598-017-18142-y.
- Huber, M.E., Chiovetto, E., Giese, M.A., & Sternad, D. (2020). Rigid foot soles improve balance in beam walking but improvements do not persist with bare feet. Scientific Reports, 10(1):7629.
- Russo, M., Lee, J-W., Hogan, N., Sternad, d. (in preparation). Postural balance with mechanical support.
- Lee, J.W., Huber, M.E., Chiovetto, E., Giese, M., Sternad, D., & Hogan, N. (2019). Human-inspired balance model to account for foot-beam interaction mechanics. IEEE International Conference on Robotics and Automation (ICRA 2019), 1969-1974, Montreal, Canada, May 20-24.
- Lee, J., Huber, M.E., Sternad, D., Hogan, N. (2018). Robot controllers compatible with human beam balancing behavior. IEEE International Conference on Intelligent Robots and Systems (IROS), Madrid, Spain, October 1-5.
- Shiozawa, K., Lee, J., Russo, M., Sternad, D., & Hogan, N. (under review). Frequency-dependent force direction elucidates neural control of balance. Journal of NeuroEngineering and Rehabilitation.
Dynamic Primitives, A Fundamental Hypothesis on Motor Control
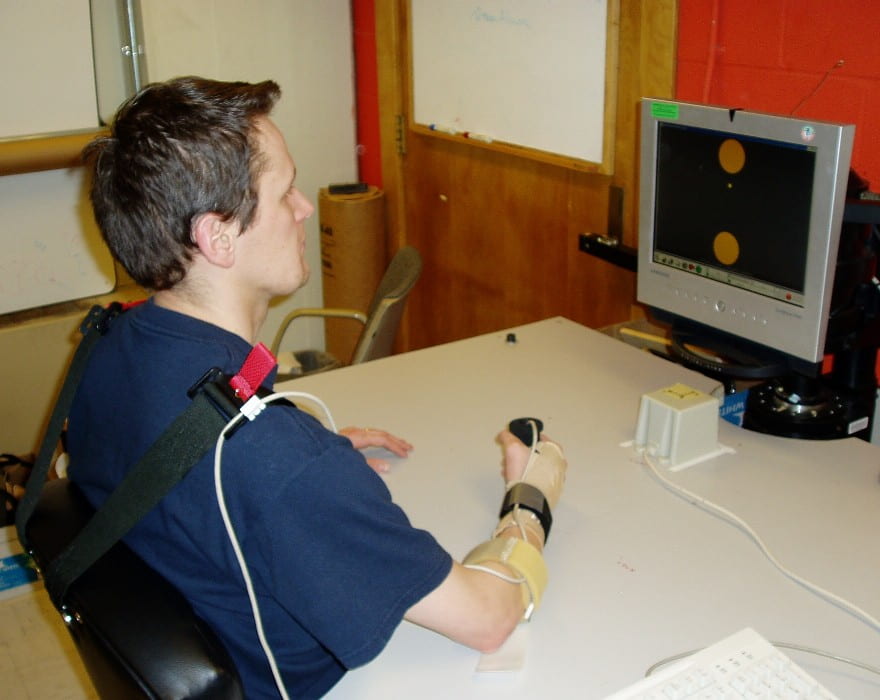
Daily activities consist of a coordinated sequence or combination of rhythmic and discrete movements. Examples range from rhythmic locomotion when it is combined with stepping over obstacles, to rhythmic finger actions in piano playing, while translating the hand over the keyboard. A longstanding question in motor control is whether such complex actions are controlled by simpler units that can be regarded as control primitives. Due to fundamental features of the neuromuscular system, most notably its slow response, we argue control may be in terms of parameterized primitives, which may simplify learning, performance, and retention of complex skills [1]. Specifically, the hypothesis is that submovements, oscillations, and impedance primitives are coupled to generate complex movements, the latter is necessary for interaction with objects and the environment [2, 3].
To test this hypothesis, we examine single- and multi-joint movements in unconstrained and interactive tasks. In one study, participants performed rhythmic movements paced by a metronome interspersed with discrete changes in their trajectory [4]; in another two studies, participants transitioned from slow to fast movements to identify constraints of these primitives and how they may be coupled [5]. Recent work examined circle drawing against a constraint showing how oscillatory primitives in joint space coupled with modeled hand impedance can account for forces against the constraint [6].
Selected Publications
- Sternad, D. (2008). Towards a unified framework for rhythmic and discrete movements: behavioral, modeling and imaging results. In A. Fuchs & V. Jirsa (Eds.), Coordination: neural, behavioral and social dynamics (pp.105-136). New York: Springer.
- Hogan, N., & Sternad, D. (2012). Dynamic primitives of motor behavior. Biological Cybernetics, 106 (11-12), 727-739.
- Hogan, N. & Sternad, D. (2013). Dynamic primitives in the control of locomotion. Frontiers in Computational Neuroscience, 7:71.
- Sternad, D. & Dean, W.J. (2003). Rhythmic and discrete elements in multi-joint coordination. Brain Research, 989, 152-171.
- Sternad, D., Marino, H., Duarte, M., Dipietro, L., Charles, S., & Hogan, N. (2013). Transitions between discrete and rhythmic primitives in a unimanual task. 2. Frontiers in Computational Neuroscience, 7:90.
- Hermus, J., Doeringer, J.A., Sternad, D. & Hogan, N. (2020). Separating neural influences from peripheral mechanics: the speed-curvature relation in mechanically-constrained actions. Journal of Neurophysiology, 123, 1870–1885.
Variability and Stability in Skill Acquisition
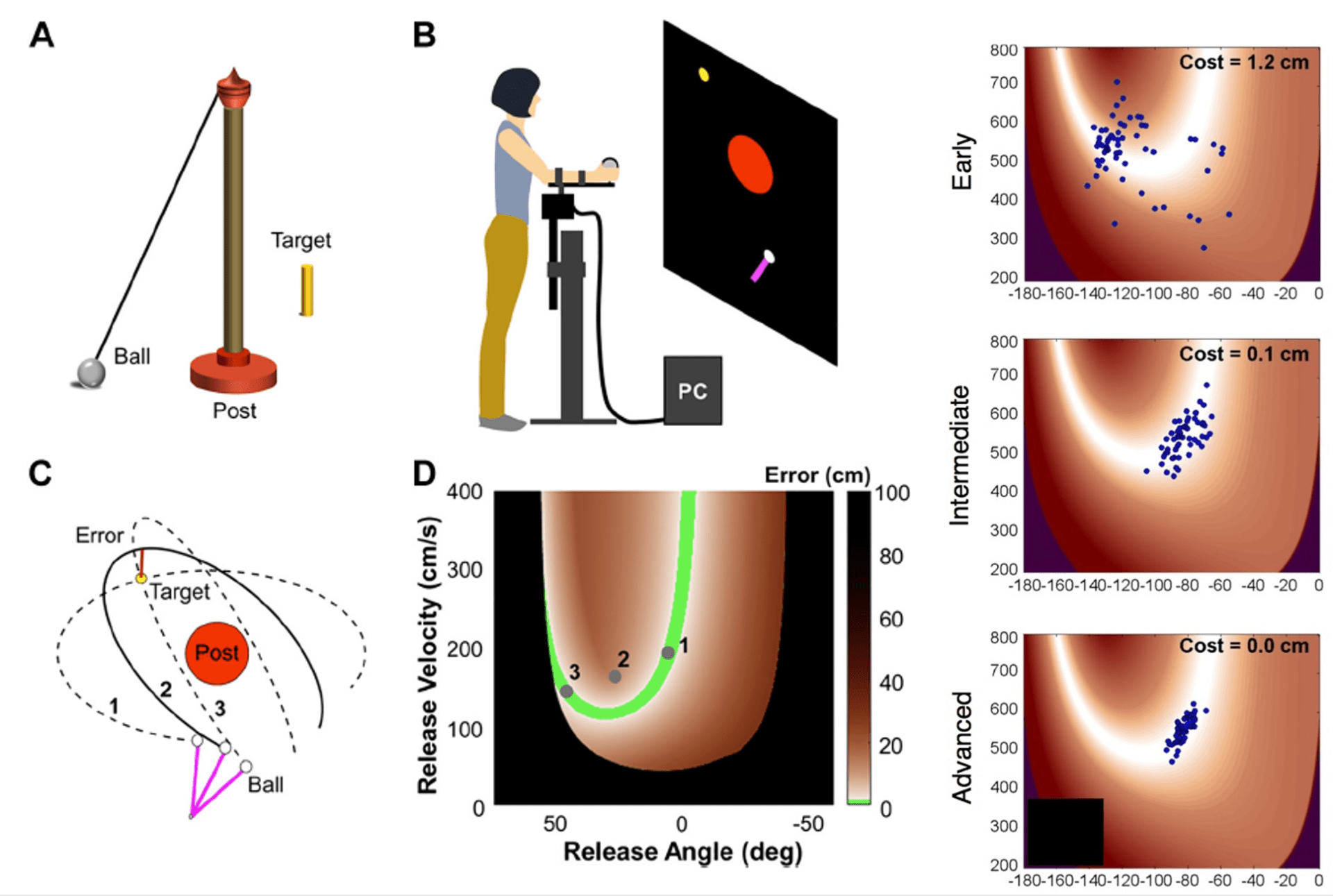
In the inquiry of acquisition and control of motor skills the concepts of stability and variability have played a central role, albeit with many different definitions. Most commonly, improvement of performance is associated with a decrease in variability of some task parameters. This reduced variability, in turn, has been interpreted as an increase in stability. This simple inverse relation obscures that empirical variability can be indicative of many different facets, ranging from the obvious “lack of control”, seen as errors in target-oriented tasks, to more beneficial aspects, such as compensatory variation between parameters, and exploration.
Dynamical stability is also a formally rigorous concept that can be quantified independently from measured variability. This research has examined skill acquisition in two selected tasks, throwing and bouncing a ball, to differentiate our understanding of variability and stability in human performance [1,2]. Recent work has focused more on skittles, a target-oriented throwing action predominantly under feedforward control. Using our method to decompose variability into three independent components: Tolerance, Noise, and Covariation (TNC-decomposition), each capturing a different contribution to successful performance [3]. Experiments test how different components of variability contribute at different stages of learning, and how stochastic noise can be a means to find successful solutions. In a recent study we also showed how performance of a sequence of discrete throws, humans unknowingly merge the successive throws into a stable rhythmic pattern. Even though the instruction was only on minimizing error at the target, subjects established a stable rhythm, as quantified by Floquet analysis [4]. Similar results were obtained in a ‘field study’, i.e., data collected in a less controlled environment of the museum of science in an outreach activity [5]. The surprising additional result of this study on ~400 participants of all ages was the female throwers were consistently less successful than male participants.
In another recent study we compared the process of acquisition in the virtual testbed and a replication of the exact task in a real setting [6]. Comparing the development of Tolerance, Noise and Covariation showed that acquisition in the virtual setting took more time and required more Tolerance than in a real task. This is noteworthy as the motor coordination in te virtual task only required a single-degree-of-freedom forearm movement, while the real throwing action involved the entire 7-dof arm. These results show that insights on leaning gained in a virtual testbed with highly controlled movements, may not ‘scale up’ to more real behaviors.
TNC Analysis Package (Matlab): Download
Selected Publications:
- Sternad, D. (2017). Human control of interactions with objects; Variability, stability, and predictability. In J.-P. Laumond, J.-B. Lasserre, & N. Mansard (eds.), Geometric and numerical foundations of movements (301-338). New York: Springer.
- Sternad, D. (2018). It’s not (only) the mean that matters: variability, noise and exploration in skill acquisition. Current Opinion in Behavioral Sciences, 20, 183-195.
- Sternad, D., Huber, M.E., & Kuznetsov, N. (2014). Acquisition of novel and complex motor skills: Stable solutions where intrinsic noise matters less. Advances in Experimental Medicine and Biology, 826, 101-124. Doi: 10.1007/978-1-4939-1338-1_8.
- Zhang, Z. & Sternad, D. (2019). The primacy of rhythm: How discrete actions merge into a stable rhythmic pattern. Journal of Neurophysiology, 121, 574-587.
- Crozier, D., Zhang, Z., Park, S-W., & Sternad, D. (2019). Gender differences in throwing re-visited: Sensorimotor coordination in a virtual ball aiming task. Frontiers in Human Neuroscience, 13, 231.
- Zhang, Z, & Sternad, D. (2020). Back to reality: Differences in learning strategies in a simplified virtual and a real throwing task. Journal of Neurophysiology, epub Nov 4. doi:1152/jn.00197.2020
Control of a Bullwhip – Human Dexterity As It Is
When you make a bed, holding a sheet (or a blanket) at its edge, how do you manage to cover the whole bed with it? Is it a single hand flick that suffices for the correct motion of the sheet or do you make an extra flick or additionally pull the sheet?
Ubiquitous in everyday life, complex objects – with multiple (infinite?) degrees of freedom, with underactuated and nonlinear dynamics – posit a puzzle for the motor neuroscience. Most previous insights have been gathered either at planar tasks involving only an arm movement or a transportation of solid objects, or when observing whole body gait. We are aiming to reveal whether the accumulated understanding of motor control can scale up to a genuinely whole-body movement together with the interaction with an underactuated, flexible bullwhip. Crack the show!
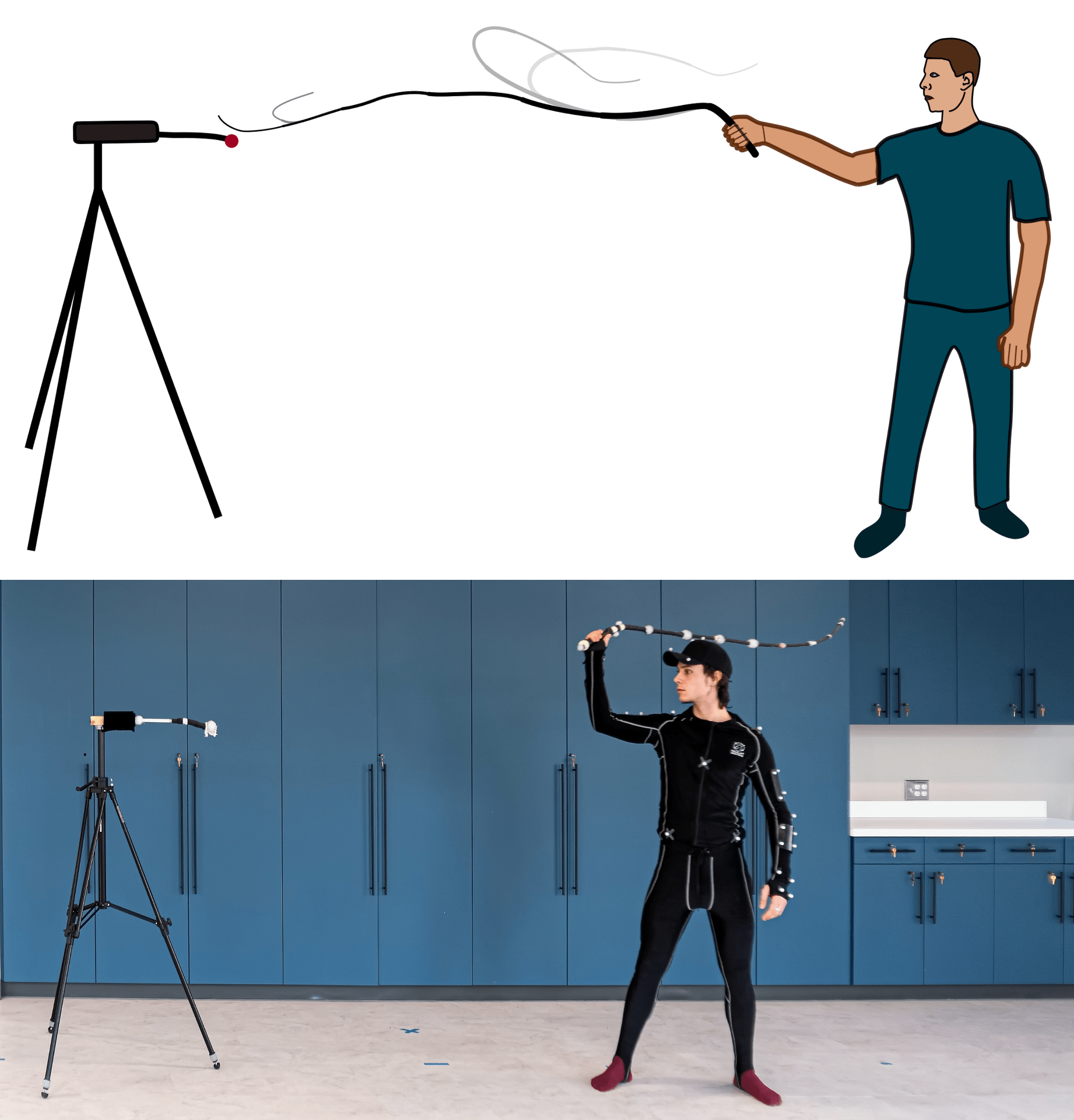
This novel paradigm, while challenging both for human participants (not as much as one would expect!) and for our researchers, opens questions of control organization, reduction of its complexity, motor learning despite nonlinearly propagating noise, modeling the flexible object dynamics, role of feedback while continuously interacting with such object and many others. Complex tasks require complex methods – so arise the novel approaches that track the body and the whip, reconstruct the kinematics and the whip continuum, describe a controller agnostic of whip dynamics and number of degrees of freedom.
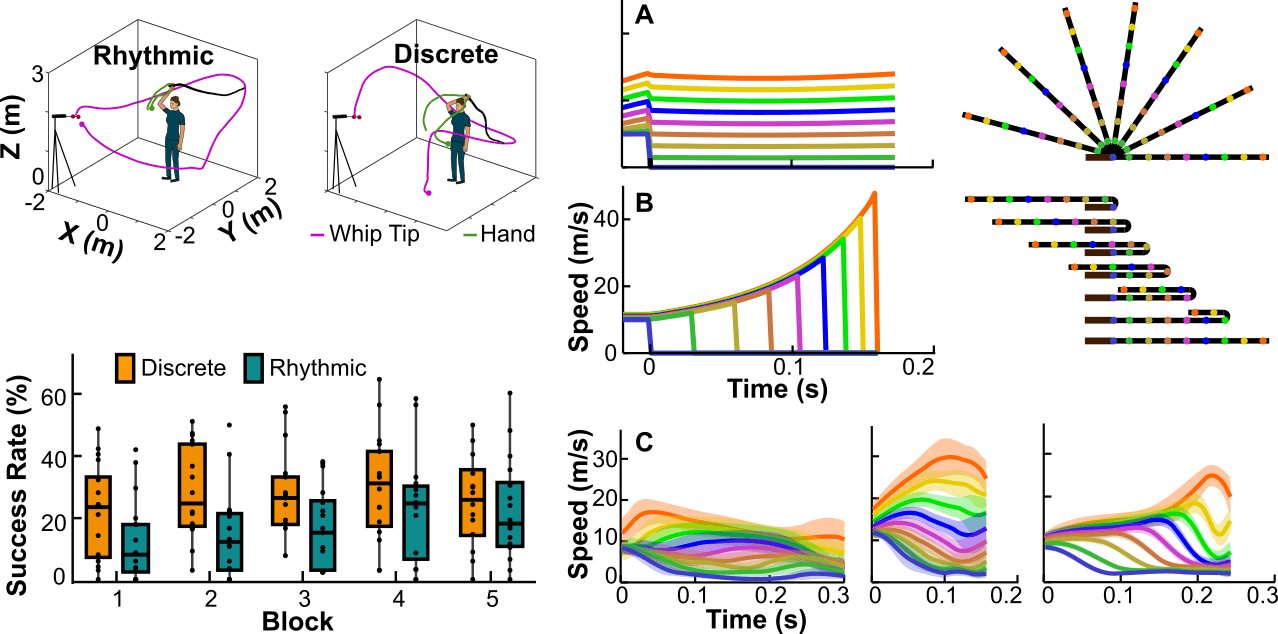
Central nervous system faces noise and delays at multiple levels as well as unprecedented number of degrees of freedom. While it is believed to rely on representation of the body’s and the environment’s physics, how complex could such representation be? What is the evidence that simple modules – dynamic movement primitives (submovements, oscillations, impedance) – simplify that representation while capturing just enough of the complex dynamics to make us as dexterous as we are? How would it change the understanding of motor control and how could it steer the development of robotics (especially ‘soft’ and ‘interaction’ areas)? This research encompasses multiple challenges faced by neuroscientists and roboticists, while inspired by an exotic (not so much just several hundred years ago!) skill of cracking the whip and by simple yet curious skill of bedmaking.
Selected Publications and Talks:
- Hogan, N., & Sternad, D. (2012). Dynamic primitives of motor behavior. Biological cybernetics, 106(11), 727-739.
- Nah M.C., Krotov A., Russo M., Sternad D., & Hogan N. (2020), “Dynamic Primitives Facilitate Manipulating a Whip,” 2020 8th IEEE RAS/EMBS International Conference for Biomedical Robotics and Biomechatronics (BioRob), 2020, 685-691, Doi: 10.1109/BioRob49111.2020.9224399.
- Krotov, A. D. (2020). Human Control of a Flexible Object: Hitting a Target with a Bull-Whip (M.S. thesis, Northeastern University)
- Nah M. C. (2020). Dynamic primitives facilitate manipulating a whip (M.S. thesis, Massachusetts Institute of Technology)
- Krotov A., Russo M., Edraki M., Sharif Razavian R., Nah M.C., Hogan N. & Sternad D. (2021) – Manipulating a Bullwhip: exploiting wave physics facilitates motor control, Neural Control of Movements (poster presentation)
- Edraki M., Sharif Razavian R., Krotov A., Russo M., Nah M.C., Hogan N. & Sternad D. (2021) – Effects of Kinematic Variability on Hitting a Target with a Bull Whip, Neural Control of Movements (poster presentation)
- Russo M., Krotov A., Edraki M., Sharif Razavian R., Nah M. C., Hogan N. & Sternad D. (2021) – Manipulating a Bull-Whip: Low-dimensional Control of an Infinite-Dimensional Object, Neural Control of Movements (panel talk)
- Xiong X., Nah M. C., Krotov A. &Sternad D., Online Impedance Adaptation Facilitates Manipulating a Whip (under review)
- Edraki M, Krotov A., Erdogmus D. & Sternad D., Modeling the complex dynamics of flexible physical systems using a NARX Network: A case study (under review)
- Nah M.C., Krotov A., Russo M., Sternad D. & Hogan N., Manipulating a whip via Dynamic Primitives – Overcoming the curse of dimensionality (under review)
- Krotov A., Sharif Razavian R., Russo M., Edraki M., Nah M. C., Hogan N. & Sternad D., Manipulating a Bullwhip – Simple Control of a Complex Object? (under review)
Movement as a Vital Sign in Preterm Infants
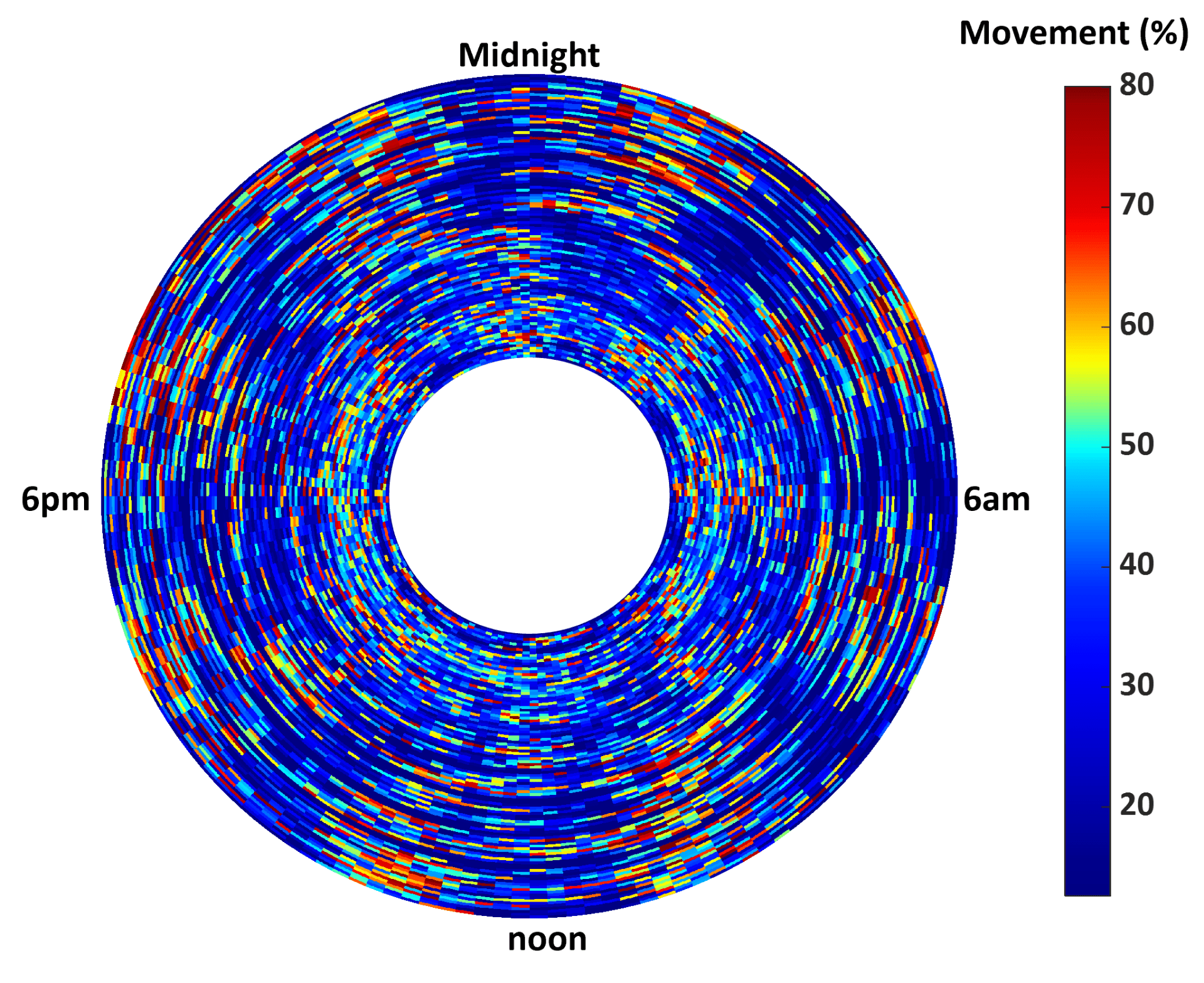
Spontaneously generated movements start in the fetus and continue to evolve to ever more complex patterns. This movement activity is the visible expression of the development of organs, the musculoskeletal apparatus, and the nervous system. Infants born preterm also provide a particularly important opportunity to study movements and their interaction with physiological function in development. These early periods provide a window of opportunity to study maturation and the development of human movements and can provide basic scientific insights. Further, preterm infants are a population at high risk given their underdeveloped physiological and nervous system. Hence, any
insights into neurotypical and atypical development will provide insights of high clinical significance. Our overarching thesis is that, like heart rate and blood pressure, movement is a vital marker for the
functional integrity of the nervous system.
Our research works with physiological data collected from large cohorts of preterm infants collected in the NICU. We analyze the routinely measured data from respiration, heart rate, and from the photoplethysmogram (PPG). The latter is the basis for the extraction of movement using our previously developed and validated algorithm that quantifies bouts of activity. Our research develops event signals, most prominently the novel extraction of movement bouts, alongside conventional metrics of inter-beat and inter-breath-intervals. This enables novel quantitative assessment of cardiovascular and respiratory activity together with movement to gain insights into early aging.
Selected Publications:
- Zuzarte, I., Sternad, D., & Paydarfar, D. (2021). Prediction of apneic events in preterm infants using cardiorespiratory and movement features. Computers Methods and Programs in Biomedicine, 209:106321.
- Zuzarte, I., Gee, A., Paydarfar, D., & Sternad, D. (2021). Effect of spontaneous movement on respiration in preterm infants. Experimental Physiology, 106, 1285-1302.
- Zuzarte, I., Gee, A.H., Sternad, D. & Paydarfar, D. (2020). Automated movement detection reveals features of maturation in preterm infants. 42 nd International Conference of the IEEE Engineering in Medicine and Biology (EMBC), Montreal, Canada, July 20-24.
- Zuzarte, I., Indic, P., Sternad, D., & Paydarfar, D. (2019). Quantifying movement in preterm infants using real-time wavelet analysis of pulse oximeter signals. Annals of Biomedical Engineering, 47, 2, 646-656.